Data Quality Management: A perfect blend of machine intelligence working in tandem with human capital, by Amit Mehta and Vivek Kesireddy
We have heard a lot about data quality, but we thought it was time to explain the value of it with some real word examples. As we all know the phrases: garbage in, garbage out, while machine learning may be the brain, the data quality is the neck. After having done data quality on thousands of wells in the US land, offshore, onshore and producing wells, we came to conclusion you must deploy smart algorithms complimented by data quality management services team (24 by 7 petroleum engineering staff) to ensure analytical results are trustworthy to act upon.
We hear lot of noise in the market about AI can magically automate and fix all your data quality issues, the more high resolution data you gather often described as volume of data the AI will fix data issues etc. while that may be true tomorrow we will share some real world cases to hopefully separate signal from the noise on why it may not be true today.
Let’s focus on one low hanging fruit case of decreasing connection times which has a quick pay off for operators. For those who may not know today, pipe connections are done daily while drilling and the old mechanism of either recording each connection and sending in pdf report is a thing of past. Today has it been replaced by smart algorithms which can based on incoming raw data calculate can calculate details of the connection i.e. slip-slip, bottom to slip, slip to bottoms as they happen.
Irrespective of data source, Rig PLC, Electronic data recorder, office WITML server/data aggregators when you are pulling high resolution data many things can go wrong between the source and destination chain with the quality of data than can alter the results which users are making decisions on i.e. in this case what is our avg. connections in any hole section instantly compared to past benchmarks?
On paper it seems easy, get the source of data, apply algorithm to calculate connections, build some smarts to cancel noise and present via visualization the connection details. Right? Unfortunately, wrong! Let us share what are some possible issues which can occur with data quality which can throw of even the best algorithms acting on their own whether in office or at the edge. The data from the rig floor seldom is accurate data and needs tailoring and correction before inputting it to any predictive analytics model for Machine learning and accurate results. Often calibration issues involving human negligence or per say unreliable equipment may pose with variegate data quality problems. Let’s discuss a specific case of raw data used to automatically calculate connections and the data quality issue it poses.
Depth Jumps
As you receive high resolution data from any data source, you will see depth jumps defined as abrupt change hole depth values during active drilling activity.
In this particular case, data Provided from EDR had hole depth jumps of around 60 to70 ft data in Surface section of this well which was affecting depths at which these connections were recorded. This resulted in offset between depths at which connections were made on rig floor to the depth which was streamed by Data recorders.
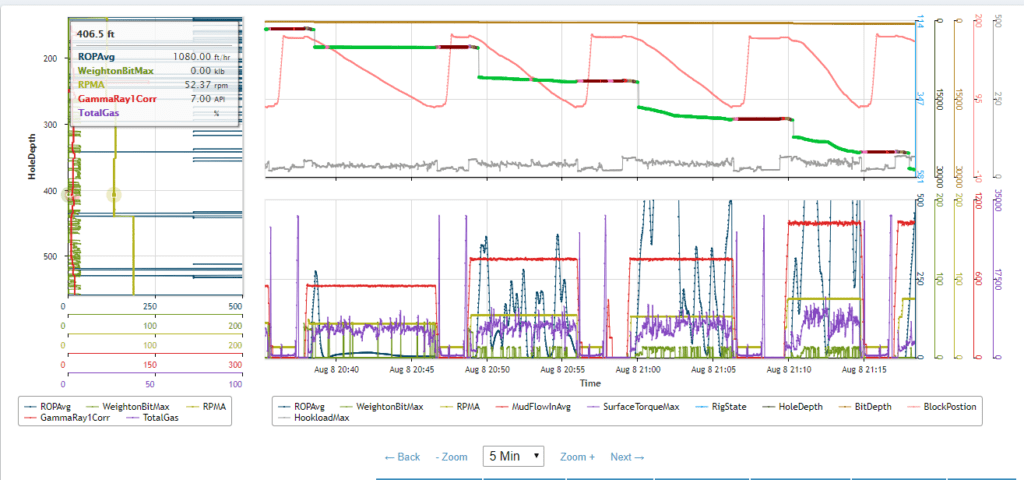
The smart algorithm could identify the depth jumps, even with some smarts fix it few times but not every time. Let’s ask why? There are multiple scenarios to it. Every depth jump might not refer to a footage gain or actual drilling activity. It might be a faulty sensor or lack of awareness of crew to calibrate sensors on sight while lowering each strand of drill pipe or a communication loss from rig floor to office environment. Consequence of any of these reasons results in incorrect connection depths being recorded and inaccurate analysis of connection times of the rig crew. A connection depth can be a great indicator of your position of bit in the hole by correlating it with depth log simultaneously.
Any predictive analysis model needs the input raw data from EDR to pass through a decision matrix or Yes/No box for any desired outcome. Since the range of communication and data quality issues have immensely greater wavelength and varying frequency of occurrence, a specific algorithm may not be able to cater for getting a smooth data curve. As a result, the outcome may be inaccurate and not reliable enough.
In this scenario, the machine itself after correcting the problem, still showed connections were made at depths mentioned below which were misleading and inaccurate.
Depth Without DQ (ft) | 295 | 409 | 508 |
Table 1.1: Connection depths before quality checks |
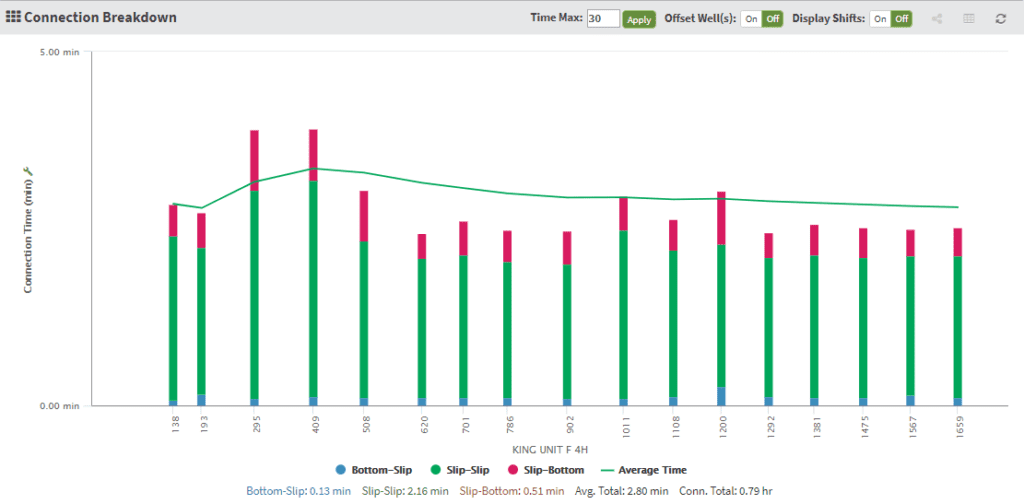
Algorithm could Identify the depth jumps due to rough increase in depth however to replicate the drilling sections and on bottom activity on a rig, human intervention is inevitable. Our RTOC data quality team experts can apply art at this point in this case helped in regaining the footage lost due to depth jumps and recalculating the depths at which connections were made. Post data quality analysis of the flagged areas of interest, the validated connection depths were found to be as mentioned below which showed that there was an average offset of 50ft before data quality operations were performed .
Depth DQ (ft) | 264 | 351 | 439 |
Table 1.2: Connection depths after quality checks
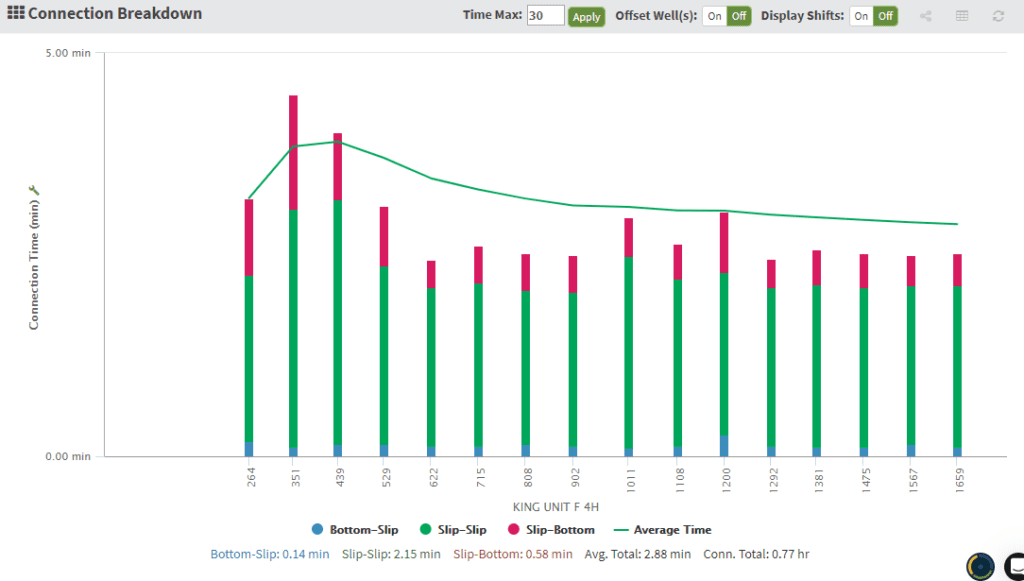
This is just one of many possible data quality issues which can affect the connection time analysis, to learn more visit us at www.moblize.com or call us